Encoding Expert Knowledge for Food Processing Technologies
People are versatile and can learn to function expertly in many different capacities, writes Dr Ai-Ping Hu from the Georgia Tech Research Institute.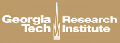
As we move forward into conceiving a poultry processing plant of the future – which will be made up of highly adaptable and scalable systems that are simultaneously capable of handling ‘lot sizes of one’ – this kind of versatility will be invaluable.
In the context of automation, the author invites the reader to envision a new breed of all-purpose robots working inside a poultry processing plant. These robots would be made of almost generic hardware (in the same way that human beings are all physiologically similar), but their control algorithms (their ‘training,’ from which expertise in using one’s five senses is derived) would be custom-tailored for accomplishing a myriad of different processing tasks. In all likelihood, some of the tasks in the poultry processing plant of the future have yet to be foreseen.

The tradition of apprenticeship is an age-old practice by which expert knowledge and skills are passed from one generation to the next. As robots are beginning to assist in increasingly sophisticated tasks that were once the exclusive domain of human practitioners (surgery, navigating in unstructured environments, complex decision making, to name just a few), there has been much research devoted to the subject of how to teach these new machines old tricks.
A new project just initiated through the Agricultural Technology Research Program at the Georgia Tech Research Institute (GTRI) will explore how expert knowledge in the food processing industry may be encoded and then used to teach robots to perform more like their human counterparts.
Though plants are increasingly automated, there remain in the food processing industry a surfeit of operations that require expert knowledge in order to function efficiently and cost-effectively. In the poultry industry, there exist at least two such operations: deboning and trimming. Taken together, these two operations tend to require the largest contingent of manual on-the-line labour inside poultry processing plants. Fixed automation solutions exist for deboning but their use in plants perennially results in a loss of yield compared to humans performing the same task.
Researchers in GTRI’s Food Processing Technology Division have been working on an Intelligent Deboning project for several years – this new research project will complement that existing project. Likewise, trimming is comprised of a number of actions that are fairly simple for human beings to perform, but that are especially difficult for machines to implement robustly, namely: visually inspecting naturally variable product and identifying regions of interest, tactile manipulation of non-rigid objects, support/fixturing and cutting of deformable material. This new project will have direct application to the support/fixturing and cutting aspect of the trimming operation.
To properly perform deboning and trimming, i.e. to do them quickly, accurately, and efficiently (with minimal energy), human practitioners undergo a period of learning, during which time they develop experience and expertise in manipulating a knife. Figure A depicts a high-level schematic of the operation.
The environment, in this case, is the bird. The input states to the human controller are made up of properties of the environment that can be sensed and measured. These are, principally, in the case of biomaterials: textural compliance (differentiating among meat, tendon, and bone) and orientation, i.e. how the bird is fixed. The control outputs include the position and orientation of the knife (three Cartesian coordinates and three angles) and the forces and torques applied to (about) the knife handle.
The input-output mappings – for a given bird (input), how does a line worker wield a knife (output)? – corresponding to especially skilled practitioners comprise a valuable fund of expert knowledge. For the new project, this expert knowledge (or finesse) will be encoded and learned by a robot attempting to take the place of the human controller in Figure A.
Two types of measurements will be required: kinematic (geometry and motion) and dynamic (forces and torques). In each case, the hand-held knife is the conduit. To make the kinematic measurements, GTRI proposes to use the Microsoft Kinect. To make the dynamic measurements, the project will use the instrumented knife test–bed utilised by the Ergonomic Work Assessment System (EWAS) project.
For the encoding and teaching, GTRI intends to explore a combined neural network and fuzzy logic technical approach. Neural networks are adept at using numerical information (e.g., data obtained from multiple sensor measurements) to drive the creation of highly non–linear, interconnected mappings. Fuzzy logic is a good choice for translating linguistic rules (such as “IF the force on the blade feels hard, THEN ease off the knife handle”) into quantifiable actions. Taken together, the two methods will (1) permit a numerical encoding of expert knowledge and (2) provide the capability for a robot to refine its performance in real time through practice, so that its actions during deboning and trimming may more closely replicate that of a human expert.
Of course, the physical embodiment of an all-purpose robot that can learn from such encodings may look quite different (and cost much less) than existing robots. GTRI researchers are tackling this complementary aspect of the problem, as well – a topic they will reserve for a future article.
Reprinted from PoultryTech, a publication of the Agricultural Technology Research Program of the Georgia Tech Research Institute, a programme conducted in cooperation with the Georgia Poultry Federation with funding from the Georgia Legislature.
February 2012